World Health Organization (WHO) reports reveal that 24% of the global burden of disease is attributed to modifiable environmental factors. Exposure to environmental pollution is associated with many diseases including Asthma, Diabetes, Cataract and other Non-Communicable Diseases (NCDs). NCDs kill 41 million people each year at present, equivalent to 71% of all deaths globally. Our research aims to
- Develop an improved methodology to pre-process environmental sensor data
- Identify the environmental profiles associated with asthma, cataract, diabetes and obesity for disease management and control
- Identify the spatial distribution (hotspots) and emerging hotspots of diseases
- Examine the impact of environmental (air quality, temperature, rainfall) factors on the mortality of NCDs
Research Projects
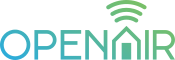
SMART solutions for Air Quality testing and promote best practices for City Councils. [SDG3, SDG6, SDG11, SDG13, SDG15] – Funded by DPIE and NSSN – 2.4 Million
Operational Network of Air Quality Impact Resources (OPENAIR) addresses the significant health issues and premature deaths caused by poor air quality in Australian communities. Current air quality monitoring in NSW is conducted by the Department of Planning and Environment (DPE) using high-precision, costly equipment. However, some local councils collect air quality data using affordable equipment, resulting in limited impact due to a lack of consensus on technology design and application. To overcome this, the objective is to leverage low-cost environmental sensors to gather localized air quality data in urban areas, road intersections, and pollution hotspots. This data aims to provide additional information to the public and serve as a resource for councils to address air quality concerns. The ultimate goal is to create an information hub that facilitates data sharing with the broader community.
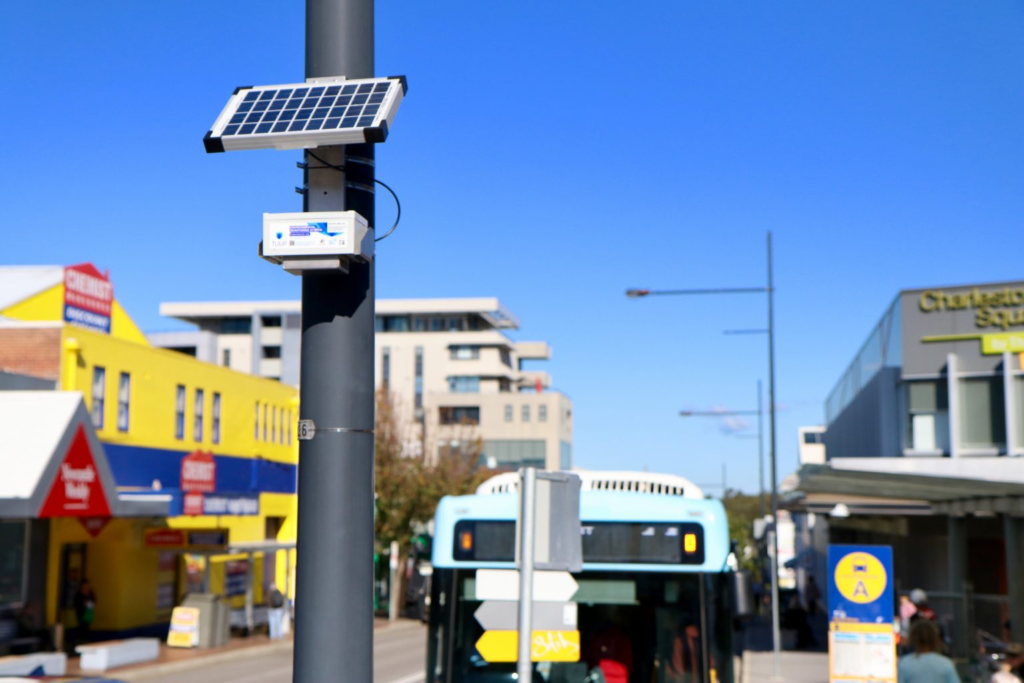
Collaborating partners include Department of Planning and Environment (DPE), NSW Sensor Network (NSSN), Participating universities, The University of Technology Sydney (UTS), Australian National University (ANU), University of Sydney, University of New South Wales (UNSW), and the University of Western Sydney and Participating City Councils, Hawkesbury, Lake Macquarie, Muswellbrook, Newcastle, Northern Beaches, Orange, Parramatta, Sunshine Coast, Sutherland, Tweed, Wollondilly and Wollongong.
Environmental Sensor Data Pre-processing by Identifying and Imputing Large Gaps of Sensor Data – to Identify Risk Factors Associated with Population Health – Funded by WSU
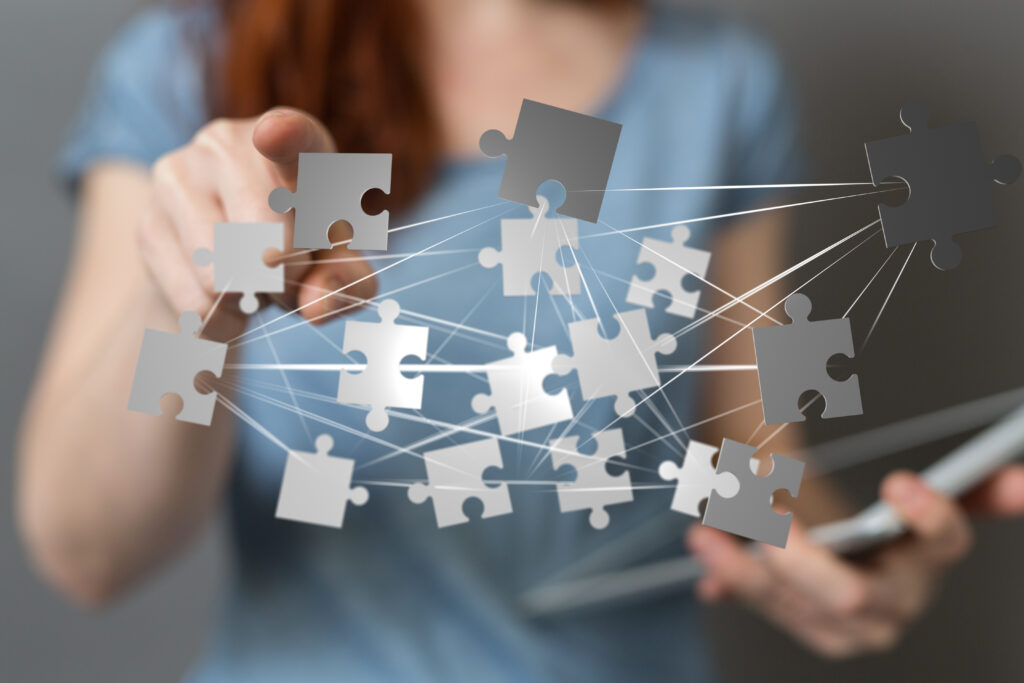
Weather and pollution data are spatiotemporal. Missing values are ubiquitous in these datasets as the data is being collected through sensors. Well-established imputation techniques are still not sufficient to deal with spatiotemporal datasets. Existing methods that deal with missing data in time series perform reasonably well in situations where the percentage of missing values is relatively low, and the gap size is small. However, the need for the development of robust methods, particularly for large gaps, is still persistent. This research develops an improved methodology to impute missing values in weather and pollution data in a spatiotemporal setting.
Identifying Environmental Profiles Associated with Cataracts for Disease Management and Control – Funded by WSU
In 2019, the WHO reported that approximately 65.2 million people worldwide have cataracts. Previous studies identified the association of environmental factors such as long-term exposure to UV radiations and air pollutants such as ground-level ozone (O3), nitrogen dioxide (NO2), Sulphur dioxide (SO2), particulate matter (PM10 and PM2.5) with cataract formation and aggravation. A better understanding of the association of these risk factors with cataract will drive towards strategies to protect eye health in future. This research identifies the environmental profiles associated with cataract by developing a Bayesian hierarchical model addressing the spatiotemporal variation of environmental and health data for disease management and control.
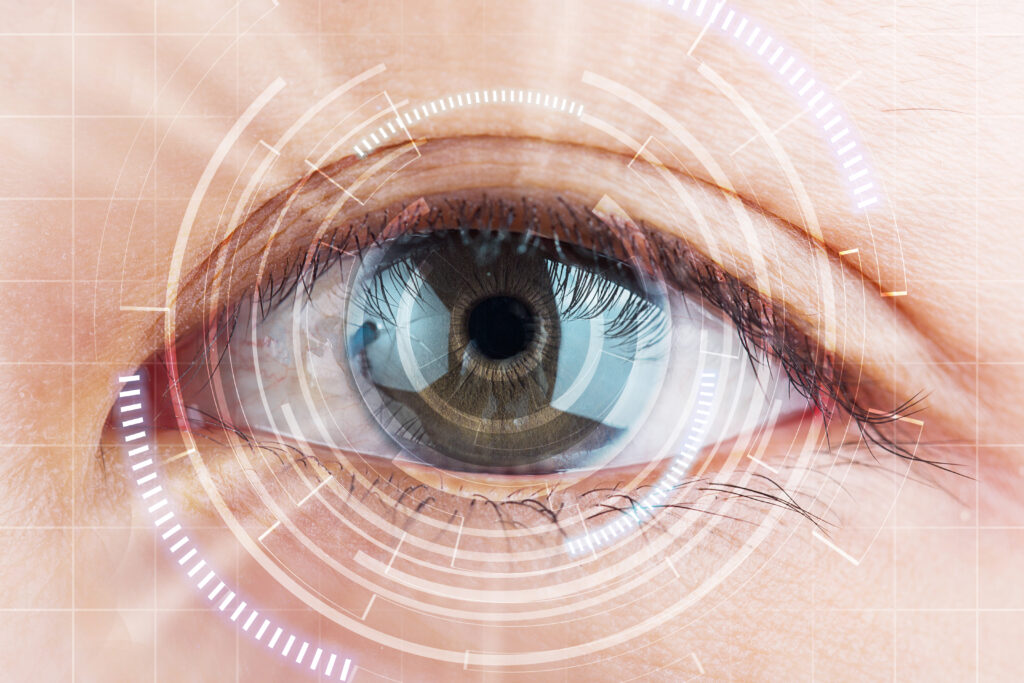
Exploring the Factors Affecting NCDs for Health Management with Special Reference to Cardiovascular Diseases, Cancers and Diabetes in Sri Lanka – Funded by AHEAD (UN-World Bank & WSU)
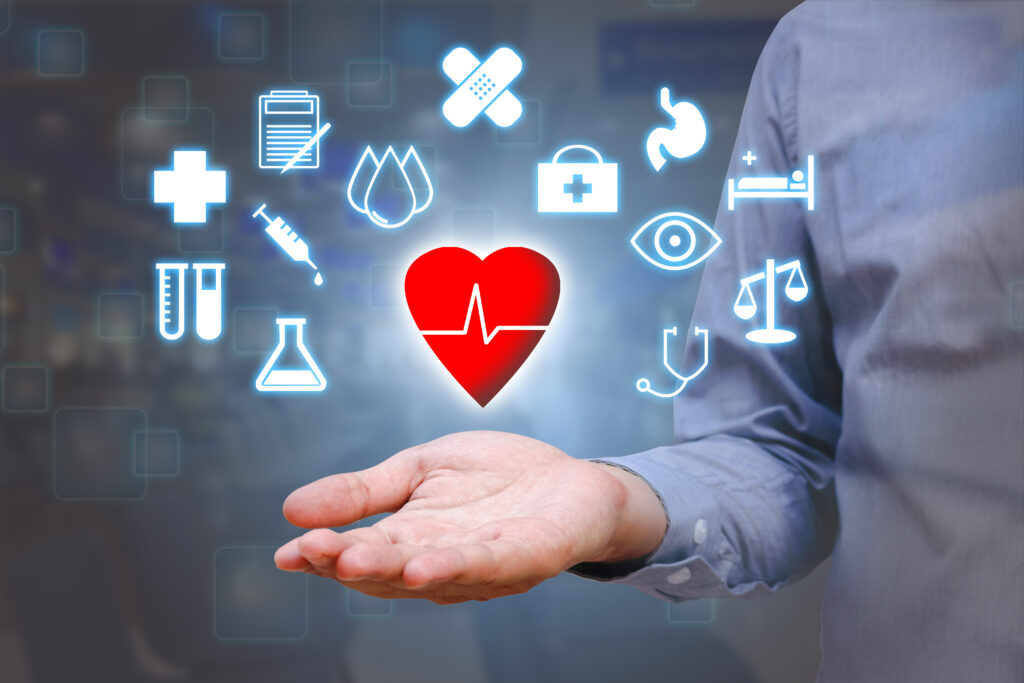
Non-Communicable Diseases (NCDs) are the major causes of morbidity and mortality worldwide, indicating an essential public health problem. Each year, more than 15 million people die from an NCD between the ages of 30 and 69 years; 85% of these “premature” deaths occur in low- and middle-income countries (WHO, 2021). Currently, chronic NCDs are overtaking communicable diseases as the dominant health problem and are now the leading causes of mortality and morbidity in Sri Lanka. This study aims to identify the trends and patterns of the most leading NCDs and to explore demographic, socioeconomic, biological, behavioural and environmental factors affecting non-communicable diseases in Sri Lanka.
Data-Driven Calibration of Low-Cost Air Quality Sensors – Funded by WSU
Air pollution poses a significant threat to environmental and public health worldwide, making the monitoring of air quality at high spatial and temporal resolutions crucial for implementing effective mitigation measures. Traditional high-end monitoring stations, while accurate, are costly and challenging to deploy densely. In contrast, low-cost air quality sensors (LCSs) offer a more affordable and practical solution, though their accuracy and reliability can be problematic. This project addresses a critical gap: the absence of effective calibration methods for LCS networks after deployment. Our aim is to overcome the obstacles associated with the post-deployment recalibration of LCS networks by developing a novel methodology that enables the transfer of calibration parameters between geographically distant static sensor networks. This research will employ machine learning and transfer learning techniques for calibration, moving beyond conventional lab-based approaches.
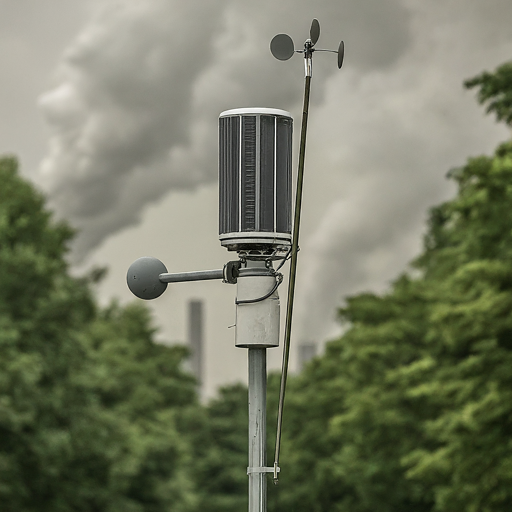
Advanced Estimation of Long-Term Air Pollutant Exposure Using Sensor Data
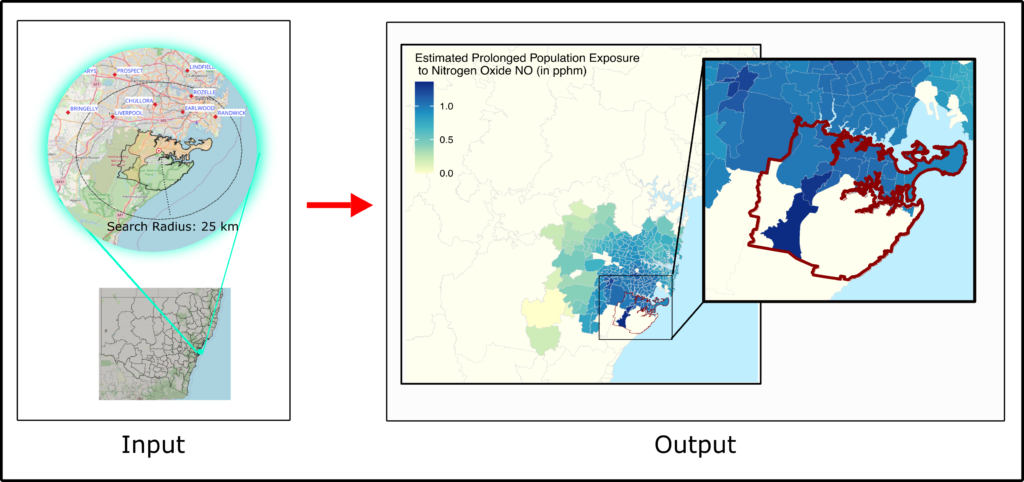
Long-term exposure to air pollutants is linked to serious health issues, including cataracts, diabetes, cancer, and cardiovascular diseases. For effective disease prevention and management, it’s essential to estimate such prolonged exposure accurately. Traditionally, sensor data have been used to monitor air pollutant levels, offering snapshots that can infer long-term trends. However, accurately estimating prolonged exposure presents challenges, notably the limited spatial distribution of sensors, which hampers precise exposure assessment. Furthermore, existing methods often focus on either detailed spatial predictions or long-term averages, neglecting the need for a combined spatial-temporal resolution.
Our project addresses these issues by proposing a new methodology to estimate prolonged exposure to air pollutants with an improved consideration of both spatial and temporal dimensions. This innovative approach not only deepens our understanding of how long-term exposure to air pollution correlates with health risks but also aids in pinpointing areas of critical air quality concern.
Video Gallery
Identifying Environmental Risk Factors Associated with Cataract for Disease Management and Control
Spatiotemporal Data Analysis to Identify Environmental Risk Factors Associated with Diabetes
Exploring the Factors Affecting Non-Communicable Diseases for Health Management
Posters
Posters presented at the Westmead Research and Innovation Conference 2022 on 18-19 August 2022 in Sydney.
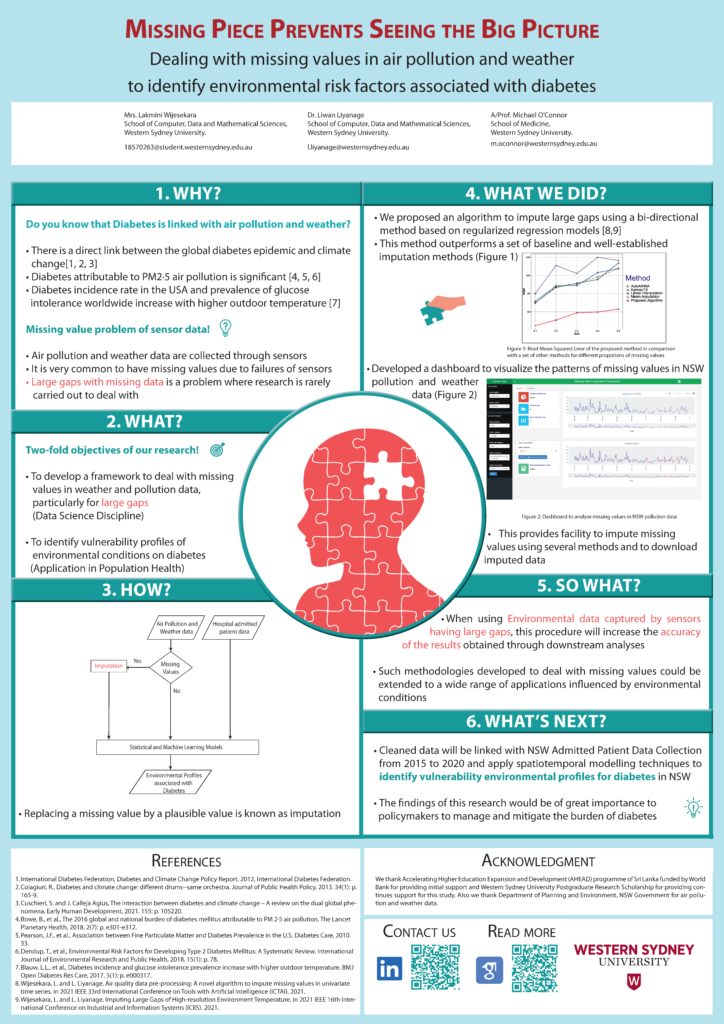
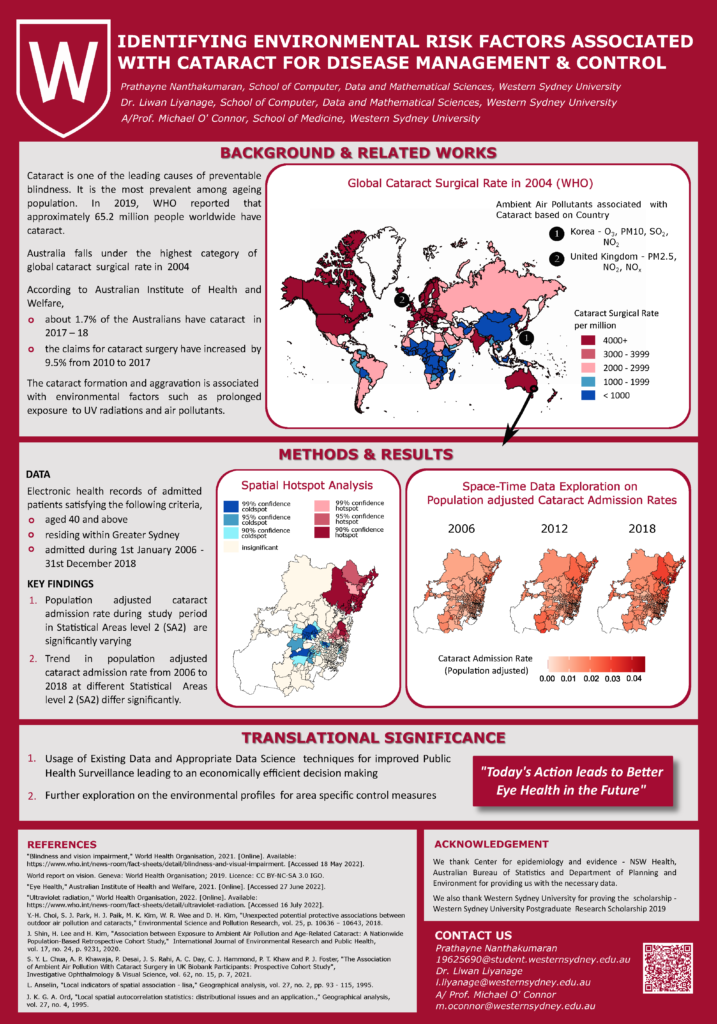
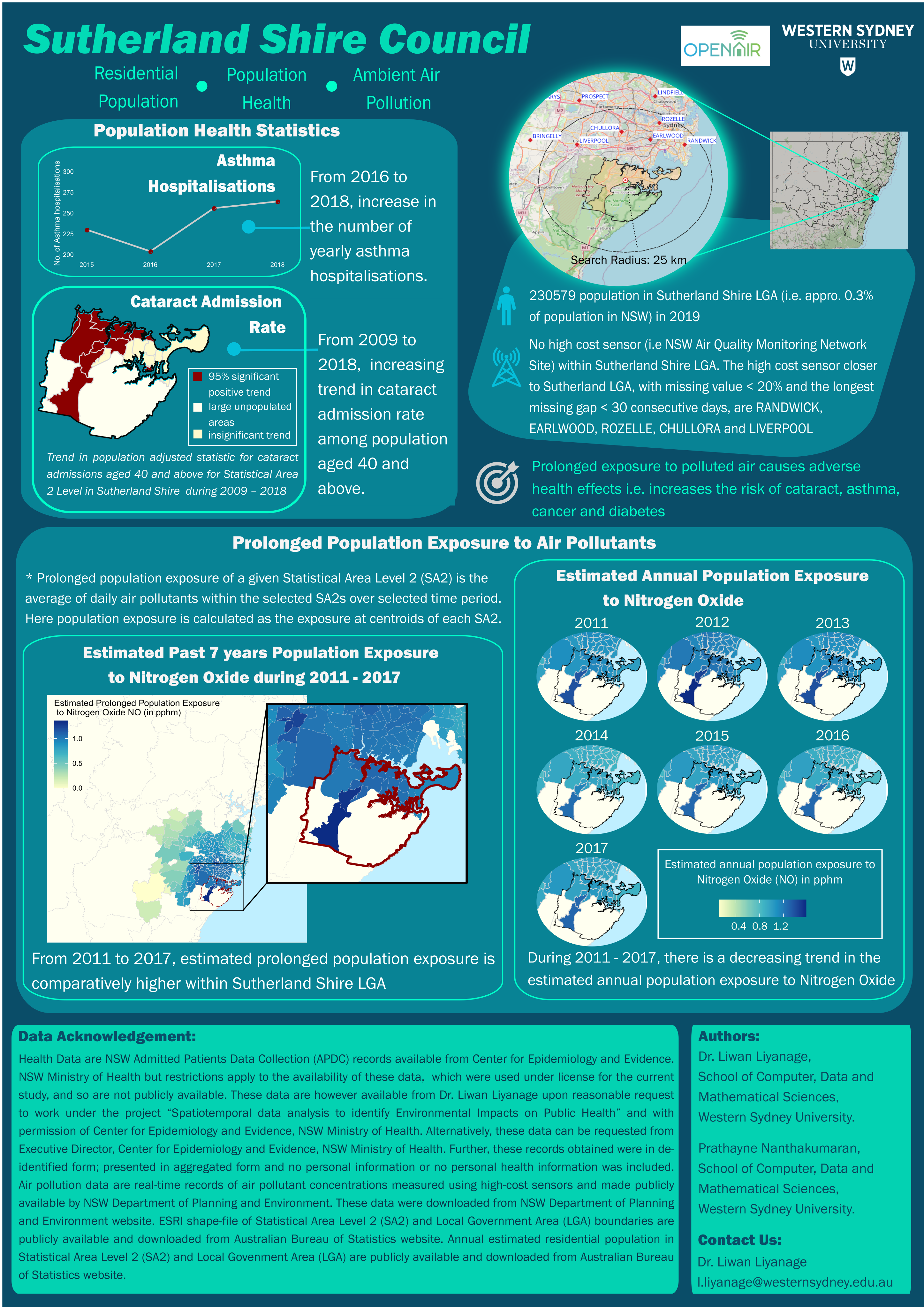
Publications
- Nanthakumaran P, Liyanage L (2024), ‘Comparison of interpolation techniques for prolonged exposure estimation: a case study on seven years of daily nitrogen oxide in Greater Sydney’, Data Science and Machine Learning: 21st Australasian Conference, AusDM 2023 Auckland, New Zealand, December 11-13, 2023 Proceedings.
- Jayarathne, H., & Liyanage, L. (2024). Gender differentials of non-communicable diseases. Population Association of America 2024 Conference. Ohio: Population Association of America.
- Jayarathne, H., & Liyanage, L. (2023). Spatial Distribution of Non-Communicable Diseases and Implications for Community Vulnerability: A Case Study in Sri Lanka, 2030 AND BEYOND: Risk-informed decision making, investment and behaviour (pp. 36-37). University of Hudders¬eld, United Kingdom
- Jayarathne, H., & Liyanage, L. (2023). Exploring the environmental factors affecting Emerging Infectious Diseases for Health Management. AUA International Conference on Public Health Resilience: Faculty of Public Health UI Science Festival Feb 2023, Jakarta Indonesia
- Jayarathne, H, and Liyanage. Gender differentials of non-communicable diseases for Health Management. Accepted for presentation at the UKADR Conference 2022 to be held on 7-8 December, Edinburgh University, United Kingdom.
- Jayarathne, H, and Liyanage. Spatial distribution of Non-Communicable Diseases for Health Management. Accepted for presentation at the 20th Australian Population Conference (APA) to be held on 23-25 November 2022 in Australian National University, Canberra.
- Nanthakumaran, P., and Liyanage, L. (2021, July). Tree-Based Models Using Random Grid Search Optimization for Disease Classification Based on Environmental Factors: A Case Study on Asthma Hospitalizations. In 2021 IEEE 2nd International Conference on Pattern Recognition and Machine Learning (PRML) (pp. 136-142). IEEE. https://doi.org/10.1109/PRML52754.2021.9520720
- Wijesekara, L., Nanthakumaran, P., and Liyanage. Space and Time data exploration of air quality based on PM10 sensor data in Greater Sydney 2015-2021. Accepted for presentation at the 15th International Conference on Sensing Technology (ICST’15) to be held on 5-7 December 2022 in Sydney.
- Wijesekara, L., and Liyanage, L. (2021, November). Air quality data pre-processing: A novel algorithm to impute missing values in univariate time series. In 2021 IEEE 33rd International Conference on Tools with Artificial Intelligence (ICTAI) (pp. 996-1001). IEEE. https://doi.org/10.1109/ICTAI52525.2021.00159
- Wijesekara, L., and Liyanage, L. (2021). Imputing Large Gaps of High-resolution Environment Temperature. In 2021 IEEE 16th International Conference on Industrial and Information Systems (ICIIS) (pp. 74-79). IEEE. https://doi.org/10.1109/ICIIS53135.2021.9660672
- Wijesekara, W. M. L. K. N., and Liyanage, L. (2020, March). Comparison of imputation methods for missing values in air pollution data: Case study on Sydney air quality index. In Future of Information and Communication Conference (pp. 257-269). Springer, Cham. https://doi.org/10.1007/978-3-030-39442-4_20
- Wijesekara, L., and Liyanage, L. (2020, November). Modelling Environmental Impact on Public Health using Machine Learning: Case Study on Asthma. In 2020 5th International Conference on Innovative Technologies in Intelligent Systems and Industrial Applications (CITISIA) (pp. 1-7). IEEE. https://doi.org/10.1109/CITISIA50690.2020.9397488